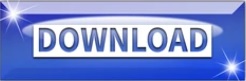
SpeCal was intensively tested to ensure the consistency of all reduced images (cADI, Loci, TLoci, PCA, and others) for any SPHERE observing strategy (ADI, SDI, ASDI as well as the accuracy of the astrometry and photometry of detected point-like sources.

SpeCal is meant to extract the astrometry and photometry of detected point-like sources (exoplanets, brown dwarfs, or background sources). Then, it uses the speckle calibration tool (SpeCal) to minimize the stellar light halo that prevents us from detecting faint sources like exoplanets or circumstellar disks.
ASTROMETRY VERSUS PHOTOMETRY ASTRONOMY SOFTWARE
First, the software corrects for instrumental artifacts. To complement the European Southern Observatory pipeline, the SPHERE consortium developed a dedicated piece of software to process the data. The large amount of data must be uniformly processed so that accurate and homogeneous measurements of photometry and astrometry can be obtained for any source in the field. The main part of this time (200 nights) is dedicated to the detection and characterization of young and giant exoplanets on wide orbits.Īims. The consortium of the Spectro-Polarimetric High-contrast Exoplanet REsearch installed at the Very Large Telescope (SPHERE/VLT) has been operating its guaranteed observation time (260 nights over five years) since February 2015. Geneva Observatory, University of Geneva, Chemin des Mailettes 51, 1290Ĭontext. Millenium Nucleus “Protoplanetary Disks in ALMA Early Science”, Valparaiso, Chile Núcleo de Astronomía, Facultad de Ingeniería, Universidad Diego Portales, Av. Universidad de Chile, Camino el Observatorio 1515, Santiago, Chile Université Côte d’Azur, OCA, CNRS, Lagrange, France Institute for Astronomy, University of Edinburgh, Blackford Hill, Edinburgh EH9 3HJ, UKĭepartment of Astronomy, Stockholm University, 106 91 Max-Planck-Institut für Astronomie, Königstuhl 17, 69117 Université Grenoble Alpes, CNRS, IPAG, 38000Īix-Marseille Université, CNRS, LAM (Laboratoire d’Astrophysique de Marseille) UMR 7326, 13388ĬRAL, UMR 5574, CNRS, Université de Lyon, Ecole Normale Supérieure de Lyon, 46 Allée d’Italie, 69364 INCT, Universidad De Atacama, calle Copayapu 485, Copiapó, Atacama, Chile Paris 06, Sorbonne Paris Cité, 5 place Jules Janssen, 92190Į-mail: – Osservatorio Astronomico di Padova, Vicolo della Osservatorio 5, 35122

Lesia, Observatoire de Paris, PSL Research University, CNRS, Sorbonne Universités, Univ.
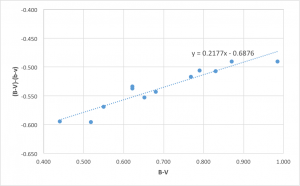
Punctuation and style concerns regarding equations, figures, tables, and footnotes The algorithm could be integrated into data processing pipelines of WFSATs to increase their response speed and detection ability to time-domain astronomical events. The algorithm proposed in this paper could obtain types, positions and magnitudes of celestial objects with high accuracy and cost around 0.125 second to process an image, regardless of its size. To reduce this effect, we propose to use transfer learning strategy to train the neural network with real data. Therefore, we further investigate the epistemic uncertainty of our algorithm and have found that differences of background noises and differences of point spread functions between the training data and the real would introduce uncertainties to final measurements. Because the photometry and astrometry neural network are data-driven regression algorithms, limited training data with limited diversity would introduce the epistemic uncertainty to final regression results. In this paper, we extend the ability of a deep neural network based astronomical target detection algorithm to make it suitable for photometry and astrometry, by adding two new branches. An automatic data processing algorithm which could detect celestial objects and obtain their positions and magnitudes from observed images is important for further scientific research. Wide field small aperture telescopes (WFSATs) are preferable observation instruments for time domain astronomy, because they could obtain images of celestial objects with high cadence in a cost-effective way.
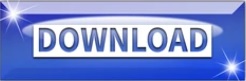